32 Introduction: The Central Limit Theorem
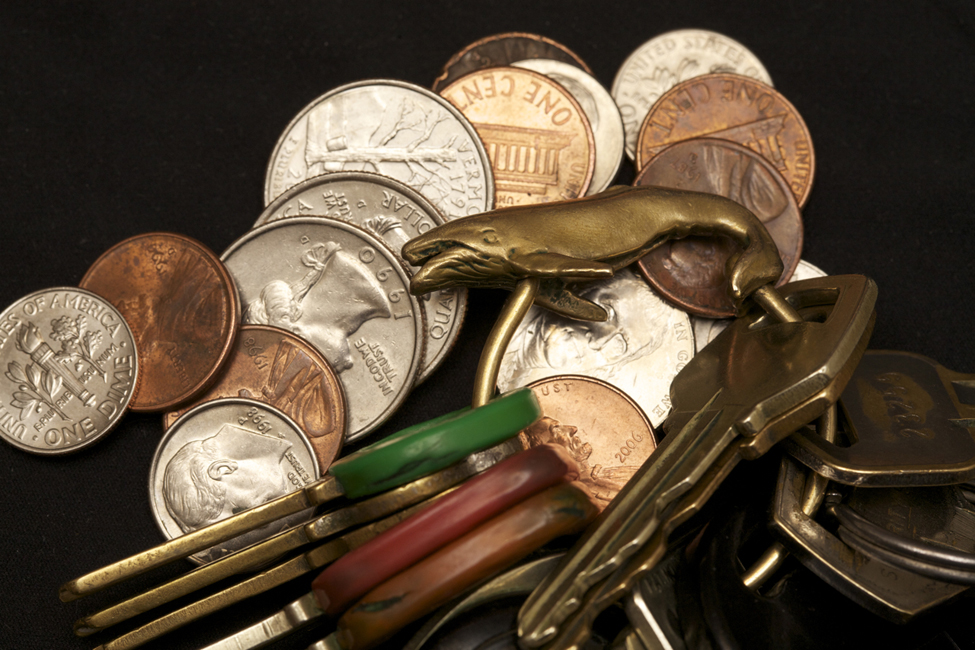
Learning Objectives
By the end of this chapter, the student should be able to:
- Recognize central limit theorem problems.
- Classify continuous word problems by their distributions.
- Apply and interpret the central limit theorem for means.
- Apply and interpret the central limit theorem for sums.
Why are we so concerned with means? Two reasons are: they give us a middle ground for comparison, and they are easy to calculate. In this chapter, you will study means and the central limit theorem.
The central limit theorem (clt for short) is one of the most powerful and useful ideas in all of statistics. There are two alternative forms of the theorem, and both alternatives are concerned with drawing finite samples size n from a population with a known mean, μ, and a known standard deviation, σ. The first alternative says that if we collect samples of size n with a “large enough n,” calculate each sample’s mean, and create a histogram of those means, then the resulting histogram will tend to have an approximate normal bell shape. The second alternative says that if we again collect samples of size n that are “large enough,” calculate the sum of each sample and create a histogram, then the resulting histogram will again tend to have a normal bell-shape.
In either case, it does not matter what the distribution of the original population is, or whether you even need to know it. The important fact is that the distribution of sample means and the sums tend to follow the normal distribution.
The size of the sample, n, that is required in order to be “large enough” depends on the original population from which the samples are drawn (the sample size should be at least 30 or the data should come from a normal distribution). If the original population is far from normal, then more observations are needed for the sample means or sums to be normal. Sampling is done with replacement.